The Complete Guide to Image Labeling Tools for Data Annotation
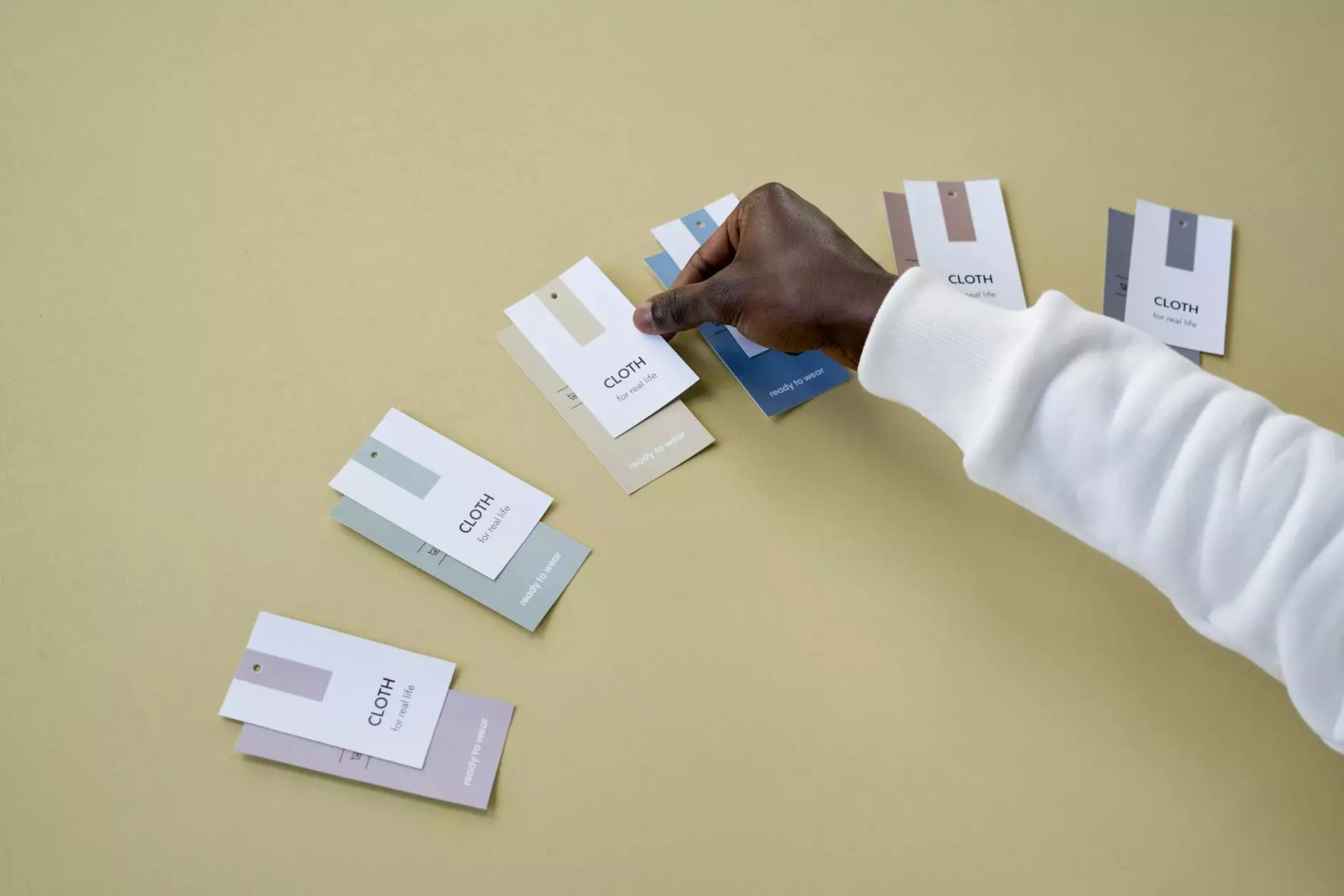
Understanding Image Labeling Tools
In the age of artificial intelligence and machine learning, the importance of image labeling tools cannot be overstated. These tools allow businesses to annotate and label images accurately, ensuring that datasets used for training AI models are of high quality. The process of image labeling involves assigning meaningful tags and categories to digital images, which enables computers to interpret and analyze visual data effectively.
Why Image Labeling is Crucial for Modern Businesses
As businesses across various industries embrace automation and machine learning, the demand for rich datasets grows exponentially. Here are several reasons why image labeling is critical:
- Enhanced AI Training: Labeling images correctly ensures AI models understand the context and nuances of visual information.
- Improved Accuracy and Efficiency: Well-labeled data leads to improved performance in image recognition tasks, reducing errors.
- Streamlined Data Management: Using image labeling tools allows businesses to organize and manage their datasets effectively.
- Competitive Advantage: Companies that leverage high-quality annotated data gain a significant edge over their competitors.
The Core Features of an Effective Image Labeling Tool
When searching for an image labeling tool, it’s essential to consider several features that can enhance your labeling process:
- User-Friendly Interface: A simple and intuitive design allows users to quickly get accustomed to the tool.
- Automation Capabilities: Automated features like pre-labeling can significantly reduce the time taken for data annotation.
- Collaboration Tools: Features that allow multiple users to collaborate on labeling projects can increase productivity.
- Integration with Other Platforms: The ability to integrate with various data management and machine learning platforms is crucial.
- Customizable Features: Custom tagging and labeling options that fit specific business needs enhance flexibility.
- Quality Control Measures: Built-in quality assurance checks can help maintain high standards in data accuracy.
Top Image Labeling Tools in the Market
With a plethora of options available, here are some of the leading image labeling tools that businesses can consider:
1. Keylabs.ai
Keylabs.ai stands out as an excellent choice for businesses focused on high-quality data annotation. With its user-friendly interface and robust features, it supports various image labeling tasks, making it perfect for training sophisticated AI models.
2. Labelbox
Labelbox is another powerful platform that offers versatile labeling options. It is known for its collaboration features, allowing teams to work together seamlessly on annotation projects.
3. Supervisely
Supervisely excels in providing tools for both image and video data annotation. It also supports machine learning workflows, making it a comprehensive solution for data scientists and developers.
4. Scale AI
Scale AI focuses on providing high-quality, human-annotated data. Their advanced workflows and automation capabilities make the image labeling process efficient and reliable.
5. VGG Image Annotator (VIA)
This open-source tool is widely used for both image and video annotation. Its flexibility in handling various tasks makes it a favorite among researchers.
How to Choose the Right Image Labeling Tool
Choosing the right image labeling tool depends on several factors. Here’s a guide to help you make an informed decision:
- Define Your Requirements: Assess your specific needs. Are you annotating images for object detection, segmentation, or classification?
- Evaluate Features: Look for tools that offer features pertinent to your requirements, such as real-time collaboration and quality assurance.
- Consider Scalability: Choose a tool that can grow with your projects and accommodate higher volumes of data as needed.
- Check Integration Compatibility: Ensure the tool integrates seamlessly with your existing software ecosystem.
- Assess Pricing: Compare costs versus the features offered. Look for a balance between affordability and functionality.
- Seek Reviews and Recommendations: Research customer reviews and seek recommendations to gauge user satisfaction.
The Importance of Data Quality in Image Labeling
In data-driven decision-making, the quality of the data is paramount. High-quality data ensures that AI models are trained effectively, resulting in reliable outcomes. Here’s why data quality is crucial:
- Reduces Errors: Poor quality data can lead to erroneous conclusions and decreased model performance.
- Enhances Trust: Accurate data boosts the credibility of AI models, fostering trust in automated decisions.
- Improves Compliance: Adherence to data quality standards ensures better compliance with industry regulations.
Integrating Image Labeling Tools with Machine Learning Workflows
To maximize the potential of image labeling tools, businesses should aim to integrate them with their machine learning workflows. Here’s how:
- Automate the Labeling Process: Utilize pre-labeling features to speed up the annotation process.
- Leverage Model Feedback: Use insights from machine learning models to refine labeling strategies and improve data quality continuously.
- Maintain a Feedback Loop: Encourage data scientists to provide feedback on the labeled data to ensure continuous improvement.
Challenges in Image Labeling and How to Overcome Them
Despite the benefits, image labeling comes with its challenges. Understanding these challenges and formulating solutions is vital for businesses:
- Time-Consuming Process: Labeling large datasets can be labor-intensive. Automated tools can help mitigate this issue.
- Maintaining Consistency: Inconsistent labeling can skew results. Implementing strict guidelines and quality checks can improve consistency.
- High Costs: Depending on the volume of data, costs may rise. Consider scalable solutions that grow with your needs.
Future Trends in Image Labeling
The landscape of image labeling is continuously evolving. Here are some future trends to watch for:
- Increased Use of AI in Labeling: AI tools are likely to play an even larger role in automating the labeling process.
- Focus on Unsupervised Learning: Techniques that require less human intervention for labeling will gain traction.
- Better Collaboration Tools: Enhanced platforms for teamwork and collaboration will emerge, streamlining the labeling process.
Conclusion
Investing in a high-quality image labeling tool is essential for businesses looking to leverage the power of machine learning. By prioritizing data quality, choosing the right tools, and staying aware of emerging trends, companies can position themselves at the forefront of innovation in an increasingly data-driven world. As the landscape changes, tools like those offered at Keylabs.ai will be indispensable in harnessing the full potential of visual data for the future.